Injecting Semantics for Explaining Link Predictions
Knowledge Graphs, despite their vast utlity, often suffer from incompleteness. This issue stems from their complex and automated construction process, the inherent difficulties in data collection, and the dynamic nature of the real-world phenomena they reflect. Addressing this issue, numerous Link Prediction models have been developed to infer missing facts. Most of the existing methods are based on Knowledge Graph Embeddings which are numeric representations of the entities and relations in a Knowledge Graph. These approaches are effective and scale on very large Knowledge Graphs. However, they are not capable of providing explanations for their outcomes. For this reasons, beyond prediction, explaining the outcomes has gained significant attention. Addressing this concern is Kelpie, an emerging and promising framework for explanation generation, which is gaining considerable attention due to its adaptability to any embedding model. Given a predicted link Kelpie extracts as explanation subsets of relevant facts, i.e. facts that have enabled the model to predict the link. Notably, Kelpie searches for explanations within the sub-graph composed of the facts related to the prediction to explain, considering both individual facts and combinations thereof. However, this thorough strategy of considering combinations of multiple facts leads to a significant challenge: an explosion in the number of candidate explanations. We want to enhance this strategy with respect to three goals, firstly we seek to decrease the number of candidate explanations, furthermore, we aim to maximize the performance in terms of explanation quality and lastly we strive to represent the explanations on different levels of granularity. To achieve these goals, we propose to inject semantics in the search strategy. We aim to compute an abstraction of the search space. Specifically, we aim at a new search space which is more compact and focused on more general patterns in order to support our research goals. In particular, we propose to extract the types of the sub-graph nodes and compute the quotient set – the set of equivalence classes - in order to merge similar nodes in a single node. Then, use such types to build the quotient graph which connects the equivalence classes with edges trying to preserve as much as possible the original structural properties. An edge in the quotient graph connects two equivalence classes of nodes in the original sub-graph. Hence we can map each edge in the quotient graph to a batch of facts in the original sub-graph. We execute the Kelpie search strategy using the quotient graph as input. Specifically, we enumerate all the edges in the quotient graph and subsequently all their combinations. This is equivalent to enumerating batches of facts in the original graph. The abstraction introduced by the quotient graph holds the potential to enhance the performance by directly focusing on general patterns. However, there is also a risk of losing valuable information and degrading performance. For this purpose we propose alternative quotient formulations with different (incremental) granularity. Finally a fact in the quotient graph leads to a dual perspective of explanation, in terms of equivalence classes or in terms of individual entities within. The three formulations and Kelpie, as a baseline, are experimentally compared to find the optimal trade-off between abstraction and quality.
CONSULTA INTEGRALMENTE QUESTA TESI
La consultazione è esclusivamente in formato digitale .PDF
Acquista
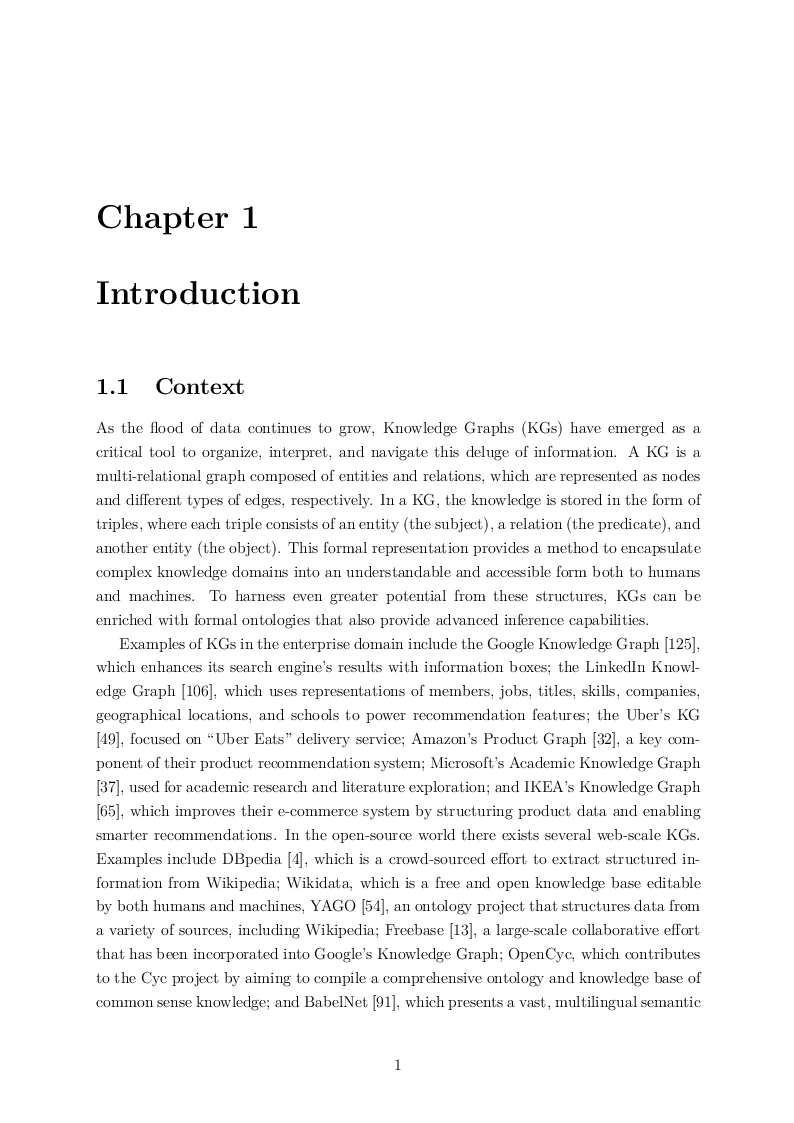
CONSULTA INTEGRALMENTE QUESTA TESI
La consultazione è esclusivamente in formato digitale .PDF
Acquista
Informazioni tesi
Autore: | Roberto Barile |
Tipo: | Laurea II ciclo (magistrale o specialistica) |
Anno: | 2022-23 |
Università: | Università degli Studi di Bari |
Facoltà: | Scienze dell'informazione |
Corso: | Informatica |
Relatore: | Claudia d'Amato |
Lingua: | Inglese |
Num. pagine: | 94 |
Forse potrebbe interessarti la tesi:
L'intelligenza artificiale ed i diritti umani
FAQ
Come consultare una tesi
Il pagamento può essere effettuato tramite carta di credito/carta prepagata, PayPal, bonifico bancario.
Confermato il pagamento si potrà consultare i file esclusivamente in formato .PDF accedendo alla propria Home Personale. Si potrà quindi procedere a salvare o stampare il file.
Maggiori informazioni
Perché consultare una tesi?
- perché affronta un singolo argomento in modo sintetico e specifico come altri testi non fanno;
- perché è un lavoro originale che si basa su una ricerca bibliografica accurata;
- perché, a differenza di altri materiali che puoi reperire online, una tesi di laurea è stata verificata da un docente universitario e dalla commissione in sede d'esame. La nostra redazione inoltre controlla prima della pubblicazione la completezza dei materiali e, dal 2009, anche l'originalità della tesi attraverso il software antiplagio Compilatio.net.
Clausole di consultazione
- L'utilizzo della consultazione integrale della tesi da parte dell'Utente che ne acquista il diritto è da considerarsi esclusivamente privato.
- Nel caso in cui l’utente che consulta la tesi volesse citarne alcune parti, dovrà inserire correttamente la fonte, come si cita un qualsiasi altro testo di riferimento bibliografico.
- L'Utente è l'unico ed esclusivo responsabile del materiale di cui acquista il diritto alla consultazione. Si impegna a non divulgare a mezzo stampa, editoria in genere, televisione, radio, Internet e/o qualsiasi altro mezzo divulgativo esistente o che venisse inventato, il contenuto della tesi che consulta o stralci della medesima. Verrà perseguito legalmente nel caso di riproduzione totale e/o parziale su qualsiasi mezzo e/o su qualsiasi supporto, nel caso di divulgazione nonché nel caso di ricavo economico derivante dallo sfruttamento del diritto acquisito.
Vuoi tradurre questa tesi?
Per raggiungerlo, è fondamentale superare la barriera rappresentata dalla lingua. Ecco perché cerchiamo persone disponibili ad effettuare la traduzione delle tesi pubblicate nel nostro sito.
Per tradurre questa tesi clicca qui »
Scopri come funziona »
DUBBI? Contattaci
Contatta la redazione a
[email protected]
Parole chiave
Tesi correlate
Non hai trovato quello che cercavi?
Abbiamo più di 45.000 Tesi di Laurea: cerca nel nostro database
Oppure consulta la sezione dedicata ad appunti universitari selezionati e pubblicati dalla nostra redazione
Ottimizza la tua ricerca:
- individua con precisione le parole chiave specifiche della tua ricerca
- elimina i termini non significativi (aggettivi, articoli, avverbi...)
- se non hai risultati amplia la ricerca con termini via via più generici (ad esempio da "anziano oncologico" a "paziente oncologico")
- utilizza la ricerca avanzata
- utilizza gli operatori booleani (and, or, "")
Idee per la tesi?
Scopri le migliori tesi scelte da noi sugli argomenti recenti
Come si scrive una tesi di laurea?
A quale cattedra chiedere la tesi? Quale sarà il docente più disponibile? Quale l'argomento più interessante per me? ...e quale quello più interessante per il mondo del lavoro?
Scarica gratuitamente la nostra guida "Come si scrive una tesi di laurea" e iscriviti alla newsletter per ricevere consigli e materiale utile.
La tesi l'ho già scritta,
ora cosa ne faccio?
La tua tesi ti ha aiutato ad ottenere quel sudato titolo di studio, ma può darti molto di più: ti differenzia dai tuoi colleghi universitari, mostra i tuoi interessi ed è un lavoro di ricerca unico, che può essere utile anche ad altri.
Il nostro consiglio è di non sprecare tutto questo lavoro:
È ora di pubblicare la tesi